The differences between sentiment analysis and emotion recognition
Which works best, when, and how using them both leads to more insight
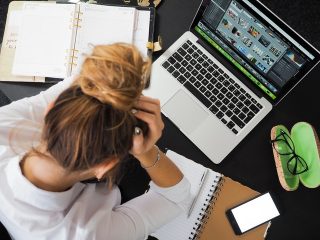
Emotions and moods are for the most part, commonly understood. Feeling an emotion may lead to a mood. Being in a mood may mean feeling certain emotions.
But when it comes to sentiment analysis and emotion recognition the lines are frequently blurred. Maybe it’s a symptom of the variety of psychological explanations of what emotions are, how they function, and what they mean. Perhaps it’s because of this that detection of sentiment, or “mood” has been operationalized and implemented into NLP/NLU, RPA, OCR and other alphabet soup projects.
Overly Sentimental
Sentiment analysis can provide you with signals that determine if a given message is “positive,” “negative,” “neutral,” or “mixed.” This gives you the mood of the message, or page, or paragraph (even over an entire volume such as a book).
Which is valuable. Determining if someone is communicating with positive, or negative frames gives the receiver an indication of the intent behind the message.
Messages contain that which is inherent in the text, what is implied by the text, and how one interprets that information. Sentiment can help to illuminate that which is implied by the text-by providing extra contextual information. These signals can determine if the message was “positive” or “negative.”
“Neutral” or “mixed” represent a problem as they are variable. How do you take action on a “mixed” signal? Is “neutral” neutral, or is the “neutral” simply an error?
Models that use sentiment analysis can’t detect emotions, can’t assist mental health patients, and if the AI model you use is trained on it the only thing you’ll get is…
Moody blues
Currently all emotion AI is a textual NLP. That means, they all run text through sentiment analysis to perform “emotion” scoring. Even those claiming to extract vocal “biomarkers” are using textual sentiment analysis…to confirm or contradict the words being spoken as illustrated in a recent article in Nature. (TL/DR: These paralanguage clues confirm text, or contradict-as in polarity. By themselves, without textual analysis, they are basically worthless at a sentence level).
Hence, the need for a textual analysis. Which for most models, means sentiment analysis (or what we like to call sentiment analysis “plus,” the plus being additional psychological scales, ML derived models, etc).
Which would be great, except that sentiment only gives you mood.
(Mood/Sentiment can still be useful-keep reading to find out how)
Read my emotions
Emotions are distinct, real for all humans, and based on neurological processes in your brain.
Things we all experience, like “love” and “anger” and “sadness.” Emotions, are Euphoric and Dysphoric, being both a “good” and “bad” feeling. Euphoric experiences encourage behavior. Dysphoric experiences dissuade behaviors too, and together they form most of the emotions…
...And then there's fear
Fear is a different beast all together. First of all, fear has been observed in a tremendous number of species throughout the animal kingdom and perhaps even in plants. It’s clear that fear is a mechanism which enables an entity to survive.
And, it’s been observed to trigger responses faster than we can comprehend it. That’s a pretty clear indication that fear is a primary force in driving behavior.
Together, these three emotional states dictate the emotions we “feel,” or experience. “Love” is euphoric. “Anger” and “Sadness” are dysphoric. We experience fear when we are “scared,” or “nervous.”
Which one works best
Emotion Recognition
Sentiment Analysis
When empathy is needed
When sympathy is needed
Emotion recognition is necessary when you want to understand what someone is feeling, not just that they are feeling something…or something positive, negative, neutral or mixed.
Sentiment Analysis is necessary when you want to understand if someone is feeling good or bad. It captures an overall mood.
Good for:
Good for:
Mental health applications, human resources, robotics, chatbots and virtual assistants, social listening, real process automation (RPA), telecommunications, customer service, marketing analysis, product reviews, and more.
Product reviews, social listening, real process automation (RPA), OCR, marketing analysis, public relations.
Example of how they can work together
“Look I don’t care if you’re sorry or not. My order should have arrived 3 days ago.”
Emotion Recognition
Emotion recognition would identify that the above statement has two sentences. The first sentence expresses anger. More sophisticated models would provide intensity or magnitude, as well as any sub-dimensional emotional signals detected like “what kind” of anger for instance. The second sentence is not emotional, but a statement of fact.
Sentiment Analysis
Sentiment analysis would average the entire message, and provide you with a “negative” detection. More sophisticated models include intensity or magnitude.
Address the feelings
Add context to the problem
With emotion recognition you and your software can understand when a person is angry and address it directly using proven methods of de-escalation. You can add a data point for any analysis, or basis for future interaction. Here, the customer is not assuaged with an apology and likely has to be escalated.
Sentiment analysis tells us that being three days late is a bad thing and leads to negative customer interactions.
Move beyond mood. Get Empathy.
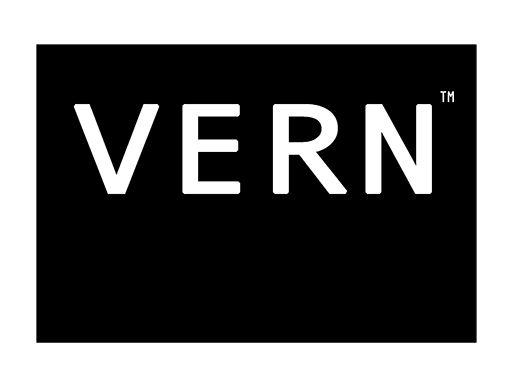
VERN™ AI is an emotion recognition, detection and prediction technology. We are emotional intelligence. Connect to us and our real-time analysis to enable your AI to empathize with your humans.